Presenter
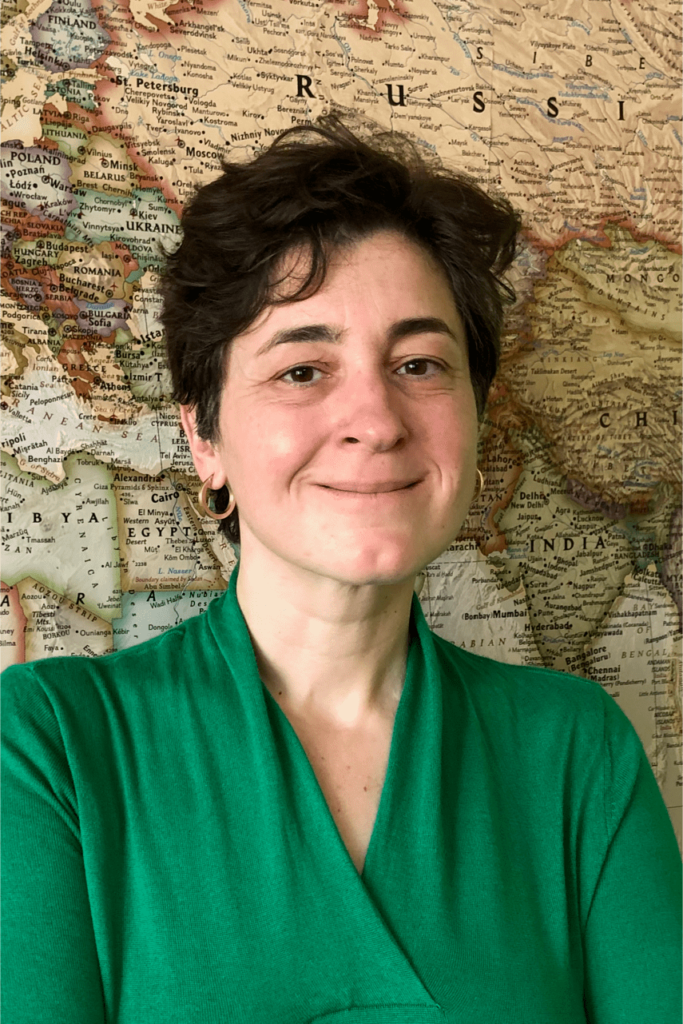
Prof. Çiğdem Gündüz Demir
Çiğdem Gündüz Demir received her B.S. and M.S. degrees in computer engineering from Boğaziçi University in 1999 and 2001, respectively, and her Ph.D. degree in computer science from Rensselaer Polytechnic Institute in 2005. She is currently a Professor of Computer Engineering and the Deputy Director of the KUIS AI Center at Koç University. Before joining Koç University, she was working as a faculty member at the Computer Engineering Department at Bilkent University. She was a visiting professor at Nanyang Technological University NTU, Singapore, in Fall 2009, and Stanford University in Spring 2013. Her main research interests and projects include development of new computational methods based on deep learning and computer vision for medical image analysis. Currently, her research group works on the interdisciplinary projects in collaborations with the Departments of Pathology and Biology for the microscopic analysis of histopathological images and in vitro fluorescence and live cell images and with the Departments of Ophthalmology and Radiology for the analysis of images acquired with in vivo imaging of CT, MR, and OCT. She was a recipient of Distinguished Young Scientist of the Turkish Academy of Sciences and CAREER Award of the National Scientific and Technological Research Council of Turkey.
Abstract
Date: April 27th, 2021 – 6:00 PM (GMT+3)
Language: English
You can register for this webinar here !